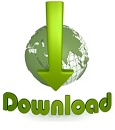


Specifically, we attempt to detect Deepfake, Face2Face and FaceSwap tampered faces in video streams. Under perioden jag arbetade på Lidl förekom allmänna butiksuppgifter så som kassabiträde, städ, varuplock och allmän kundservice. The results show state-of-the-art classification accuracy of 99.96, 99.10, and 91.20 for no, easy, and hard compression factors, respectively. To this end, we first address the under-constrained problem of facial identity recovery from monocular video by non-rigid model-based bundling. Efter studenten fick jag en anställning på Lidl, dock sa jag upp mig när jag kom in på högskolan för att bara fokusera på det. The performance is evaluated on the publicly available FaceForensics dataset. We thereby distill the best strategy for combining variations in these models along with domain specific face preprocessing techniques through extensive experimentation to obtain state-of-the-art performance on publicly available video-based facial manipulation benchmarks. Our goal is to animate the facial expressions of the target video by a source actor and re-render the manipulated output video in a photo-realistic fashion. Recurrent convolutional models are a class of deep learning models which have proven effective at exploiting the temporal information from image streams across domains. Despite the predominant effort of detecting face manipulation in still images, less attention has been paid to the identification of tampered faces in videos by taking advantage of the temporal information present in the stream. Implementation of machine learning and deep learning algorithms with ScikitLearn, ScikitImage, OpenCV and TensorFlow Machine Learning process implementation: Data Extraction, Data Exploration. The spread of misinformation through synthetically generated yet realistic images and videos has become a significant problem, calling for robust manipulation detection methods.
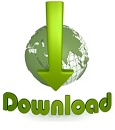